What is the ecological fallacy and how does it impact our understanding?

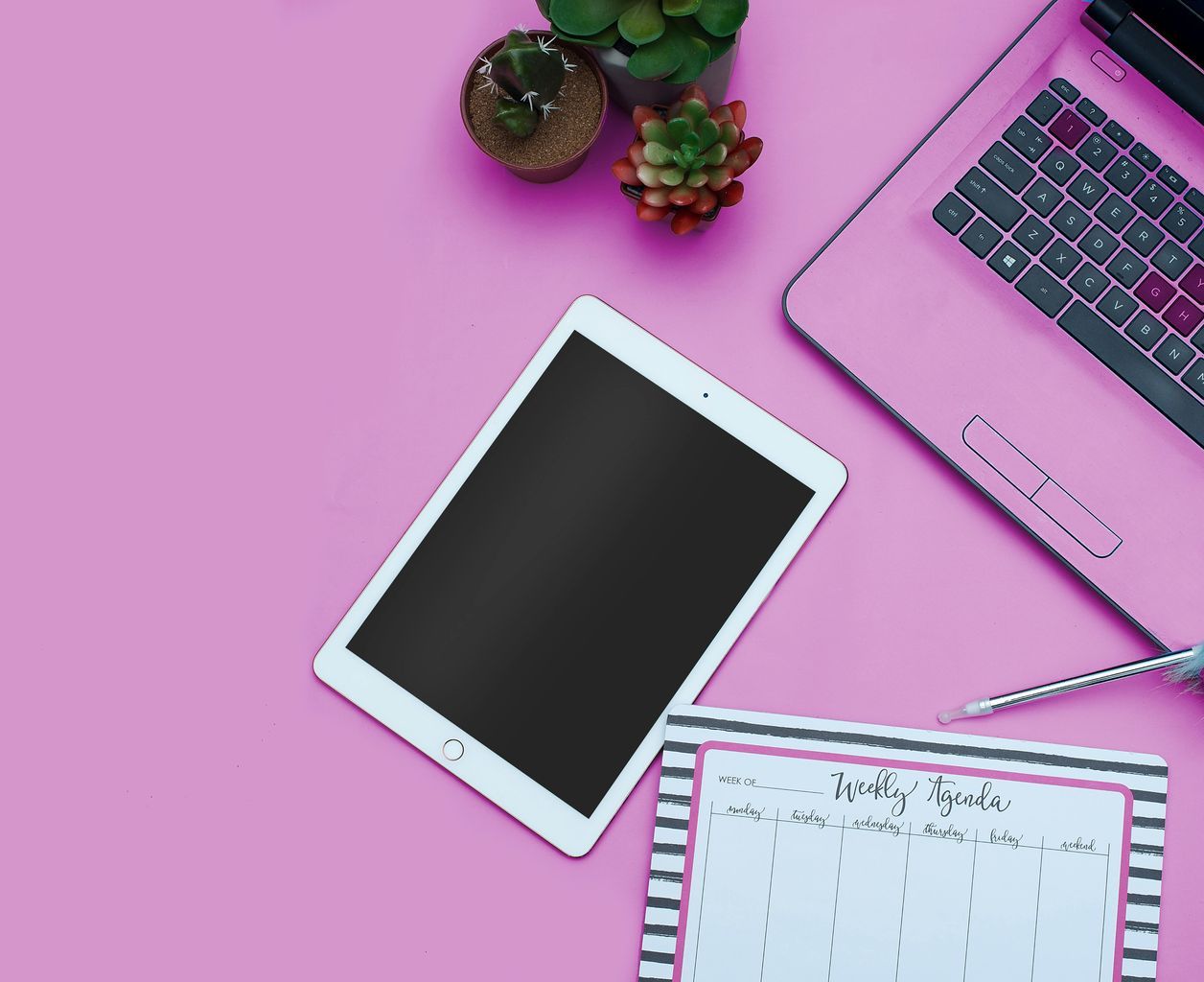
Our understanding of data can sometimes be misleading. The ecological fallacy is when we assume things about individuals based on group data. This can impact how we see social issues and public policy decisions. Knowing about this helps us be smart about information and choices. Exploring the ecological fallacy can show us how it affects our view of the world.
Definition of Ecological Fallacy
Explanation of the concept
Ecological Fallacy happens when assumptions about individuals are made from group data. For instance, studying how neighborhood wealth relates to health issues like stroke or prostate cancer using only group data could result in wrong conclusions on individual correlations.
It's important to differentiate between individual and group data to avoid Ecological Fallacy. Individual data focuses on specific points for each person, while group data looks at averages or totals for a group. Analyzing relationships accurately and avoiding Ecological Fallacy is possible by using individual-level data.
Comparing mean and median in the context of Ecological Fallacy is essential. The mean, influenced by extreme values, may skew the data and lead to misleading conclusions. On the other hand, the median, not affected by extreme values, offers a more accurate picture of the center of the data set, helping to reduce errors in ecological inference.
Distinguishing between individual and aggregate data
When conducting research, it's important to distinguish between individual data and aggregate data. This helps to avoid the ecological fallacy.
For example, when studying the relationship between voting patterns and income at the neighborhood level, individual-level data gives more accurate results than aggregate data.
Researchers like Robinson and Richards have shown that analyzing individual data leads to a better understanding of relationships between variables. For instance, looking at individual data can reveal the link between wealth and health outcomes such as prostate cancer or stroke. On the other hand, using aggregate data might oversimplify these relationships and result in incorrect policy decisions.
In a study on racial diversity in classrooms, analyzing individual correlation data is more reliable than ecological correlations. This approach provides a clearer picture of how diversity impacts the academic performance of students. In social science research, using individual data sets instead of group-level data is crucial. This helps prevent ecological inference errors, ensuring that research findings are trustworthy and valid.
Comparison of mean and median
When analyzing data sets, researchers use the mean and median to understand the central tendency of the data.
The mean is the average value, calculated by summing up all values and dividing by the total number of observations.
The median is the middle value when data points are ordered from smallest to largest.
In cases of skewed data due to outliers, the mean can be heavily influenced. In such cases, the median is a more appropriate measure of central tendency.
For example, in a study on income levels, extreme wealth or poverty can significantly impact the mean income. The median income provides a clearer picture of typical earnings.
The mean is preferred for variables that are normally distributed or symmetrical, as it considers all data points equally.
For instance, when studying classroom environments and their effect on student performance, mean scores can help identify the average academic achievement level.
Understanding the differences between the mean and median is important for making accurate inferences in research.
By knowing when to use each measure, researchers can avoid errors in statistical analysis and ensure the validity of their findings.
Examples of Ecological Fallacy
Real-life instances
In real-life situations, the ecological fallacy can be observed when researchers analyze data from groups. For example, neighborhoods or classrooms. They then make inferences about individuals based on that data.
For instance, in a study on prostate cancer and income levels, using aggregate data may suggest a correlation between wealth and the likelihood of developing prostate cancer.
However, upon closer analysis at the individual level, this relationship may not hold true. This discrepancy in findings highlights the potential error in making assumptions about individuals based on group-level data.
Correlations in real-life situations can be misleading because they can lead to the ecological fallacy. This occurs when relationships observed at the group level do not necessarily translate to the individual level.
For example, a researcher analyzing the relationship between racial diversity in a neighborhood and stroke mortality rates may find a correlation.
Making policy decisions based on this ecological correlation without considering individual data can result in flawed conclusions.
It is important for researchers to be aware of the bounds of ecological correlations. They should ensure that their data analysis considers both group and individual-level data to avoid the ecological fallacy.
Correlations and how they can be misleading
Correlations between variables can sometimes lead to misleading conclusions, especially with aggregate data.
In social science research, the ecological fallacy can happen. This occurs when making assumptions about individuals based on group-level data.
Factors like neighborhood characteristics, income levels, or racial diversity can affect the relationship between variables such as stroke mortality rates or voting patterns.
Researchers need to be careful when drawing conclusions from data at different levels.
It's important to consider individual-level data rather than just relying on group averages or descriptions.
For example, a study on prostate cancer and wealth may show an ecological correlation. But this might not be true for individual correlations.
Using regression models, like the Robinson or Richards model, can help provide more accurate inferences. These models can help avoid errors in understanding the relationship between variables at different levels.
This approach ensures a more detailed understanding of the data and helps avoid the downsides of the ecological fallacy.
Impact on Human Ecology
Understanding human behavior within ecosystems
The concept of ecological fallacy impacts our understanding of human behavior. It shows the challenges of interpreting data at individual and population levels.
For example, in voting behavior research, income may correlate with voting at the individual level. But at the group level, other variables like neighborhood diversity can change this relationship.
This discrepancy, called ecological fallacy, stresses the need to consider both individual and group data for accurate conclusions about human behavior in ecosystems.
Legal applications of the ecological fallacy affect decision-making in human ecology. For instance, in healthcare policy development, errors can arise.
In a study on prostate cancer mortality rates, individual income correlation may differ from the group level. This could lead to mistakes in policy decisions.
By using data from both individual and group levels, researchers can create better models and solutions for understanding human behavior in ecosystems.
Legal applications of the ecological fallacy
The ecological fallacy can impact legal decisions.
Researchers sometimes analyze data at group levels, like neighborhoods or income brackets. They might then make assumptions about individuals within those groups.
This can pose challenges in legal matters as relationships between variables at a group level may not apply to every individual in that group.
For instance, in a study on prostate cancer mortality rates, a correlation was found between income levels and mortality when looking at groups. However, this correlation did not hold up when examining individual data.
Differentiating between individual and group data is important in understanding the ecological fallacy in legal contexts.
Legal decisions must rely on precise individual-level data rather than generalizations from group-level studies.
In legal settings, recognizing data characteristics and the risk of errors in ecological inferences is crucial for making well-informed and fair judgments.
Challenges in Ecological Inference
Robinson's paradox and its implications
Robinson's paradox is about the limits of using overall data to draw conclusions about specific correlations in a group. This paradox shows how mistakes can happen when looking at data from a group level without also considering data from individual levels.
For instance, let's say we're studying the link between income and health, such as cancer or stroke rates. If we only look at average income data, we might make errors due to group correlations. This problem becomes bigger when we use these findings to make policies, as individual-level connections may not match up with group patterns.
To tackle this issue, researchers can turn to regression models and individual survey data to correct mistakes in group-level conclusions and find more precise links. By grasping the characteristics of the data and setting limits on their analysis, researchers can lessen the impact of Robinson's paradox. This can offer more dependable insights for making informed decisions in social science studies.
Total averages vs. individual data points
When analyzing data, it's important to distinguish between individual data points and total averages. Relying only on total averages could lead to overlooking the variability within a population, resulting in inaccurate conclusions.
For instance, in a study on wealth and health outcomes, using aggregate data might suggest a connection between higher income neighborhoods and lower mortality rates. However, analyzing individual-level data could reveal health disparities among individuals in the same neighborhoods.
Understanding these distinctions is crucial for making informed decisions in fields such as policy making and social science research. The ecological fallacy, where group-level data is incorrectly applied to individuals, can have significant consequences due to misinterpreting data.
By considering both individual and aggregate data, researchers can develop more precise models and prevent errors in statistical analysis like Simpson's Paradox.
Focusing solely on total averages without individual data can lead to inaccurate correlations and flawed inferences. By integrating individual-level data into analysis, researchers can gain a better grasp of variable properties, leading to more accurate insights and relationships.
Simpson's Paradox and its Formal Problem
Definition and explanation
Ecological Fallacy is when conclusions about individuals are made using group-level data. This mistake happens when findings about a whole population are wrongly applied to individuals in that population.
For example, in a voting behavior study, this fallacy can occur if researchers assume that because a neighborhood mostly supports a specific party, all individuals in that neighborhood also support that party.
It's important to distinguish between individual and group data to understand the ecological fallacy. Individual data focus on specific traits or behaviors of each person in a group, while group data look at overall trends or averages.
When dealing with the ecological fallacy, it's important to know that the mean is the average value of a dataset, while the median is the middle value when the data is sorted from smallest to largest.
In social science research, the ecological fallacy can impact policy decisions. For instance, drawing conclusions based on group correlations rather than individual correlations when studying health outcomes like prostate cancer or stroke can lead to inaccurate policy suggestions. Researchers need to be careful when making inferences at different levels to avoid the problems of the ecological fallacy.
Choosing the right level of analysis
When conducting research, choosing between individual or aggregated analysis greatly impacts the accuracy and validity of findings.
Analyzing data at the individual level helps identify specific correlations and relationships among variables. For example, studying the connection between income and health outcomes like prostate cancer on an individual basis reveals nuanced details.
On the other hand, examining data at an aggregate level, such as neighborhood income levels and mortality rates, offers a broader perspective but may miss important individual-level correlations.
To prevent the ecological fallacy, where conclusions from group-level data don't apply to individuals in the group, researchers should carefully select the analysis level that best fits the research question.
Using statistical models like regression analysis or ecological inference helps make accurate inferences without committing the error of ecological correlations.
Researchers should also consider the data's descriptive properties and establish clear distinctions between individual and group-level inferences to ensure the study's results' validity.
Wrapping up
The ecological fallacy happens when conclusions about individuals are made from group-level data, which can be wrong.
It can cause misleading assumptions about individuals in that group, affecting our understanding.
This may skew perceptions and result in incorrect generalizations.
Being mindful of the ecological fallacy is crucial. We should also think about individual differences when analyzing data at a group level.